목록인공지능/코세라 머신러닝 특화과정 (19)
juuuding
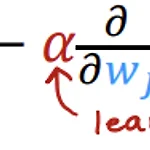
Advanced Optimization 기존 gradient descent보다 더 나은 방법으로 빠르게 loss를 최소화할 수 있는 방법에 대해 알아보자. [Gradient Descent] 아래는 간단하게 w에만 경사하강을 적용하는 식이다. alpha 값이 적절하게 설정되어 있지 않을 때 발생할 수 있는 문제에 대해 두가지 경우로 나누어 알아보자. ① alpha(learning rate)이 작을 때 learning rate이 너무 작다면 경사하강을 진행할 때 한 방향으로 아주 조금씩 움직이며 최소 loss값에 천천히 가까워진다. 이때 learning rate 값을 적절하게 높여주면 조금 더 빨리 최소 loss 값에 가까워질 수 있다. ② alpha(learning rate)이 클 때 learning ra..
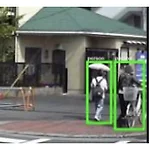
Classification with multiple outputs [Multi-label Classification] 우리가 판별해야 할 물체가 여러개 있다고 가정하자. 예를 들어 아래와 같이 사진에서 car, bus, pedestrian이 있는지 판별해야한다고 가정하자. 이러한 문제를 해결하는 방법 중 간단한 방법은 완전히 다른 머신러닝 문제로 해결하는 것이다. 예를 들어 nn1은 car을 감지, nn2는 bus 감지, nn3는 pedestrian 감지를 하는 것이다. 하지만 이는 좋지 않은 방법이다. 따라서 이러한 문제를 다음과 같이 하나의 neural network 결과로 나오게끔 해결해 볼 것이다. 이것을 multi-label classification이라고 하며 이는 multiclass clas..
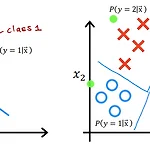
Multiclass 이때까지 y 값이 두개인 binary classification을 다루었다. 이와 달리 multiclass classification은 y가 두개 이상의 가능한 값을 가질 수 있는 것을 말한다. 아래의 그래프로 예를 들자면 왼쪽 그림은 binary classification이고, 오른쪽 그림은 y가 1,2,3,4 값을 가질 수 있는 multiclass classification이다. Softmax softmax는 binary classification인 logistic regression algorithm의 일반화이다. softmax를 사용해서 앞서 본 multiclass classification을 해결할 수 있다. 우선 2개의 결과 값을 가질 수 있는 logistic regress..
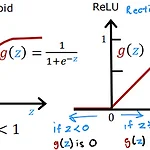
Alternatives to the sigmoid activation 이때까지 활성화 함수로 sigmoid 함수를 사용해왔다. 하지만 다른 활성화 함수를 사용하면 조금 더 강력한 neural network를 만들 수 있다. [Demand Prediction Example] 상품의 수요를 예측하는 예제로 생각해보자. unit2의 awareness 값을 안다/모른다와 같이 binary하게 나누지 말고 "the probability of awareness"를 측정하여 0 이상의 값으로 결과를 내고자 하면, a1_2의 activation function을 sigmoid가 아닌 ReLU로 설정하면 된다. ReLU에서는 0과 z 값 중 가장 최대의 값을 결과 값으로 선택하기 때문에, ReLU를 적용한 g(z)는 항..
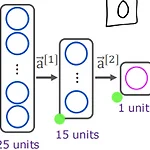
TensorFlow implementation [Train a Neural Network in TensorFlow] import tensorflow as tf from tensorflow.keras import Sequential from tensorflow.keras.layers import Dense # step 1) specify the model model = Sequential([ Dense(units=25, activation='sigmoid'), Dense(units=15, activation='sigmoid'), Dense(units=1, activation='sigmoid') ]) from tensorflow.keras.losses import BinaryCrossentropy # ste..
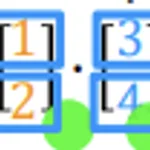
How neural networks are implemented efficiently neural network를 for loop와 vectorization을 사용하여 수행할 수 있다. 아래의 코드에서 보다시피 vectorization이 코드가 더 간결하고, 속도가 더 빠르기 때문에 vectorization을 사용하는 것이 더 좋다. [For loops] x = np.array([200,17]) W = np.array([1,-3,5], [-2,4,-6]]) b = np.array([-1,1,2]) def dense(a_in,W,b): units = W.shape[1] a_out = np.zeros(units) for j in range(units): w = W[:,j] z = np.dot(w,x) + b..
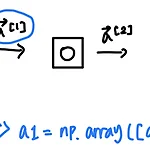
Forward prop in a single layer 앞서 본 coffee roasting 예제로 forward propagation 과정은 아래와 같은 그림으로 표현된다. # inupt data x = np.array([200,17]) 우선 layer1의 unit 1 처리 과정이다. w1_1 = np.array([1,2]) b1_1 = np.array([-1]) z1_1 = np.dot(w1_1,x)+b1_1 a1_1 = sigmoid(z1_1) 위와 마찬가지로 layer1의 unit 2, unit 3 처리 과정이다. # layer1 unit2 w1_2 = np.array([-3,4]) b1_2 = np.array([1]) z1_2 = np.dot(w1_2,x)+b1_2 a1_2 = sigmoid(z..
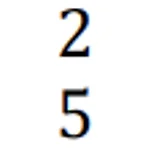
Inference in Code [Coffee roasting 예제] training set X에 temperature & duration 입력하여 good coffee / bad coffee 를 구분해보자. neural network의 layer 개수는 2개, layer1은 unit 3개, layer2는 unit 1개로 이루어져 있다고 가정한다. 그 후 layer2를 거친 값인 a2가 임계값 0.5 이상이면 "good coffee", 0.5 미만이면 "bad coffee"로 구분한다. model 생성을 위해 TensorFlow를 사용한다. # layer1 적용 x=np.array([[200.0, 17.0]]) layer_1 = Dense(units = 3, activations = 'sigmoid') ..